Imagine that public meeting that explodes in heated argument. You've likely seen the same thing online. Learn about tools for analyzing online public engagement.
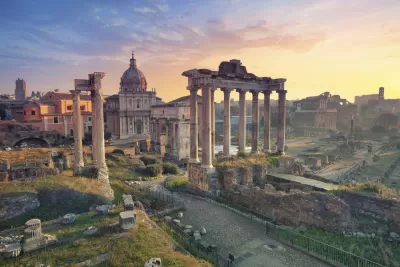
You have likely been in a public meeting that went badly and erupted into a shouting match. And sometimes the same thing can happen online too. Recently a listserv that I participate had such an eruption, resulting in hundreds of messages presenting different positions. In the case, the situation escalated very quickly and the end result was a significant portion of the group choosing to leave the listserv. When everything was over there seemed to be significant confusion how the issue escalated so quickly and why the resulting action was so dramatic.
As planners, we often find ourselves in situations where we are hosting or facilitating online participation. It can sometimes be difficult to understand how to manage online dialogue. When dialogue becomes extensive it can begin to become challenging to analyze and interpret the dialogue to extract meaning.
I use this recent example to demonstrate a handful of analysis tools. I used Linguistic Inquiry and Word Count (LIWC) software (available for $80 or $10 as a 30 day rental), which allows users to analyze any sample of text. The software is looking at the literal use of words and the psychology behind them. In this case I took all of the messages that were posted on a particular topic and assembled them into a word document. This was then imported into LIWC for analysis. The scores are tied to linguistic categories and one is comparing the results to the averages that one would see in other forms of dialogue, such as blogs, natural speech, media etc. What I am looking for is when there is a substantial difference between what one would see in typical speech or writing and what was said in the dialogue being analyzed.
- High focus on first person plural (we) and low focus on first singular, second, and third person. This indicates the people participating were writing as if they were speaking for the group.
- Very high focus on drives, due to high scores in power, achievement, and affiliation. This is the result of the conversation being dominated by people with seniority.
- A very low score for question marks. This indicates that as people were writing that they were not asking questions or seeking input from others.
- There were very low scores on perceptive processes, with low scores on every measure including see hear, feel. This indicates that people were giving their position rather than seeking to understand others positions.
One could conclude that this was a situation where participants were talking at each other, rather than truly engaging each other. A not uncommon situation in heated dialogue.
In this particular situation, there was a second dialogue of interest. While one group was engaged on a listserv, a portion of the members of the listserv were having a conversation about the listserv via a Facebook group. Think of this as the play by play sidebar. In a public meeting you could imagine it as a group at the back talking amongst themselves about what's happening at the front of the room.
If the amount of text you are capturing is limited, it can be copied from Facebook and pasted into a Word document, which can then be uploaded into LIWC for analysis. If you are trying to capture a larger portion of text, it is possible to scrape text from a Facebook group using a browser plugin called NCapture. This allows for text from a Facebook group to be imported into NVivo, a qualitative analysis software.
For the purposes of this example, I just used LIWC. When I looked at the sidebar conversation, it offers clues into what was going on. Similar to the listserv dialogue:
- There was a high focus on the use of 1st person plural (we) and low focus on first person singular, second and third person. This indicates that people were writing as if they were speaking for the group.
- There were very high scores on drives, but unlike on the listserv, where these scores were driven by high scores in power, achievement and affiliation, the high scores on Facebook were driven exclusively by affiliation. This is due to participants in the discussion focusing on their affiliation with this smaller group.
- Like the listserv, there were very low scores for question marks. Indicating that people were not seeking to ask others for input. They were making statements.
- The scores were low on perceptive process (but not as low as on the listserv), driven by low scores on see, hear, and feel. This indicates community members were giving their position rather than trying to understand others positions.
Unique to the dialogue were
- High scores on positive and negative emotion. This is not surprising since those in the discussion were reacting to what was being stated on the listserv.
- High scores on cognitive processes, due to high scores on insight, discrepancy, and differentiation. This is indicative that the group was trying to make sense of what they were reading.
- There were low scores on assent. Meaning that those participating in the discussion were not in agreement.
In a nutshell, the Facebook group was trying to figure out whether they should respond to the dialogue on the listserv, and if so, how they should respond. It’s important to remember in participatory processes that there are different participants motivated by different interests. And each participant will act in different ways, some speaking up early with the first thing on their mind, while others will step back consult with others before offering a response.
Using these tools the dialogue can be divided in any number of ways. For example, you could create a text sample that separates out participants based on their perspective on an issue, by day, or by any other measure one might want. In my example, members of the group perceived that the issue was tied to young versus old. I can look and see if indeed that is the case. I took the text sample and separated it and ran the two text samples. The results showed that the younger people showed positive emotion. They were focused on insight, causation and differentiation, but posted their remarks with tentativeness.
There are also a variety of sentiment analysis tools that allow you to put in text and it will tell you the overall sentiment found in the text as a whole. For example, this web tool and this very simple tool only look at positive, neutral, or negative sentiments. In this example, the analysis of the text sample shows that there is negative sentiment. There are many different sentiment analysis software tools available for purchase that allow for analysis of all kinds of text samples, including those that come from online participation platforms.
While these tools can help in analyzing participation after the fact, facilitation and moderation are key in any online forum. New Zealand offers helpful advice on managing online discussion forums. Chris Haller offers helpful advice for planners considering online facilitation.
Do you have tools that you use to help analyze results of online participation? Do you have best practices you use for facilitation or moderation of your online participation processes that you use when conversations become heated? Share what you are doing in the comments section.
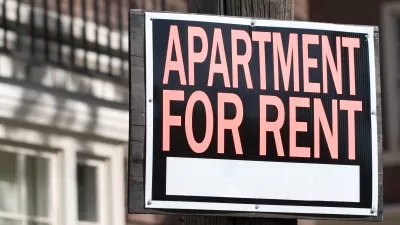
Trump Administration Could Effectively End Housing Voucher Program
Federal officials are eyeing major cuts to the Section 8 program that helps millions of low-income households pay rent.
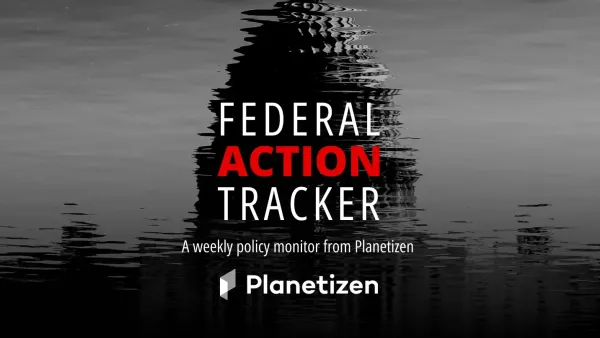
Planetizen Federal Action Tracker
A weekly monitor of how Trump’s orders and actions are impacting planners and planning in America.

Canada vs. Kamala: Whose Liberal Housing Platform Comes Out on Top?
As Canada votes for a new Prime Minister, what can America learn from the leading liberal candidate of its neighbor to the north?
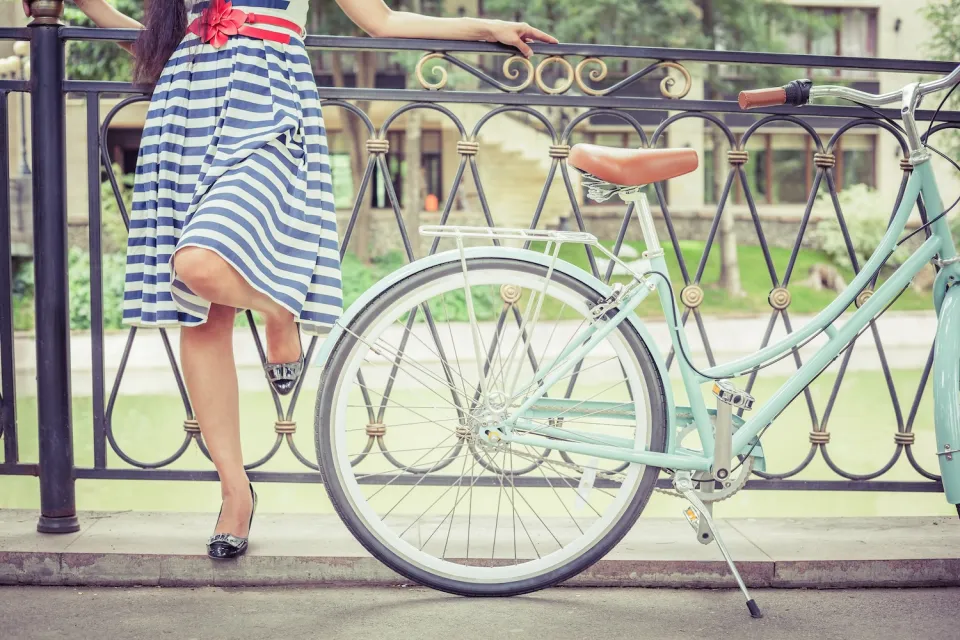
Paris Voters Approve More Car-Free Streets
Paris Mayor Anne Hidalgo says the city will develop a plan to close 500 streets to car traffic and add new bike and pedestrian infrastructure after a referendum on the proposal passed with 66 percent of the vote.
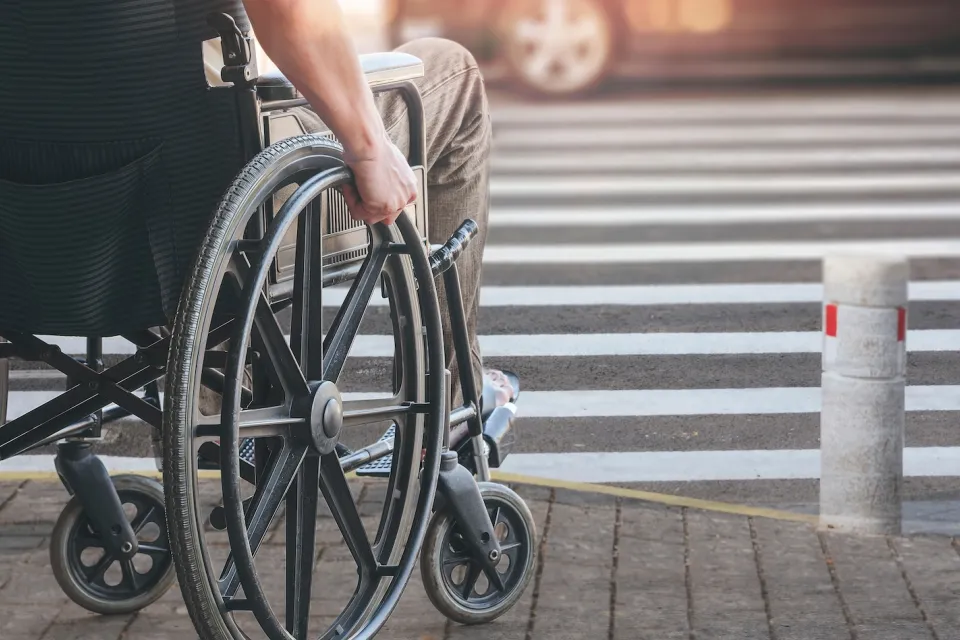
Making Mobility More Inclusive
A new study highlights the challenges people with disabilities continue to face in navigating urban spaces.
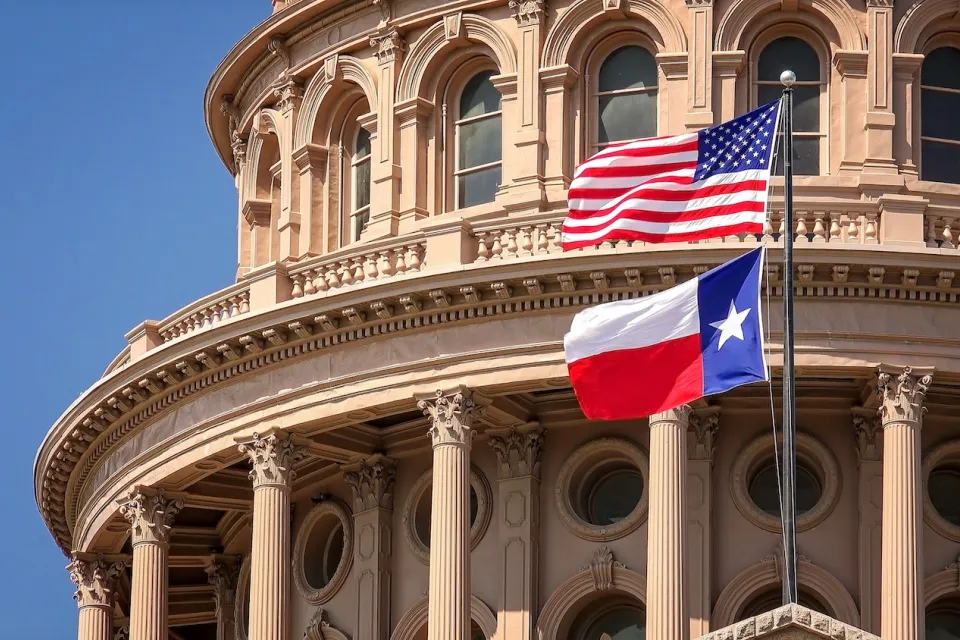
Texas Bills Could Push More People Into Homelessness
A proposal to speed up the eviction process and a bill that would accelerate enforcement of an existing camping ban could make the state’s homelessness crisis worse, advocates say.
Urban Design for Planners 1: Software Tools
This six-course series explores essential urban design concepts using open source software and equips planners with the tools they need to participate fully in the urban design process.
Planning for Universal Design
Learn the tools for implementing Universal Design in planning regulations.
Heyer Gruel & Associates PA
Ada County Highway District
Institute for Housing and Urban Development Studies (IHS)
City of Grandview
Harvard GSD Executive Education
Toledo-Lucas County Plan Commissions
Salt Lake City
NYU Wagner Graduate School of Public Service
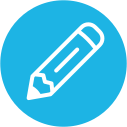